mirror of
https://github.com/rspeer/wordfreq.git
synced 2024-12-23 17:31:41 +00:00
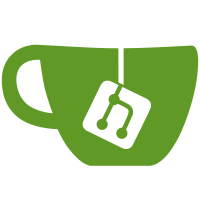
This changes the version from 1.4.2 to 1.5. Things done in this update include:
* include Common Crawl; support 11 more languages
* new frequency-merging strategy
* New sources: Chinese from Wikipedia (mostly Trad.), Dutch big list
* Remove kinda bad sources, i.e. Greek Twitter (too often kaomoji are detected as Greek) and Ukrainian Common Crawl. This results in dropping Ukrainian as an available language, and causing Greek to not be a 'large' language after all.
* Add Korean tokenization, and include MeCab files in data
* Remove marks from more languages
* Deal with commas and cedillas in Turkish and Romanian
Former-commit-id: e6a8f028e3
118 lines
4.7 KiB
Plaintext
118 lines
4.7 KiB
Plaintext
# This defines the rules on how to build parts of the wordfreq lists, using the
|
|
# Ninja build system:
|
|
#
|
|
# http://martine.github.io/ninja/manual.html
|
|
#
|
|
# Ninja is available in the 'ninja-build' Ubuntu package. It's like make with
|
|
# better parallelism and the ability for build steps to produce multiple
|
|
# outputs. The tradeoff is that its rule syntax isn't full of magic for
|
|
# expanding wildcards and finding dependencies, so in general you have to
|
|
# write the dependencies using a script.
|
|
#
|
|
# This file will become the header of the larger build.ninja file, which also
|
|
# contains the programatically-defined dependency graph.
|
|
|
|
# Variables
|
|
JQ = lib/jq-linux64
|
|
|
|
# How to build the build.ninja file itself. (Use the Makefile to get it the
|
|
# first time.)
|
|
rule build_deps
|
|
command = python -m wordfreq_builder.cli.build_deps $in > $out
|
|
|
|
# Splits the single file $in into $slices parts, whose names will be
|
|
# $prefix plus a two-digit numeric suffix.
|
|
rule split
|
|
command = mkdir -p $$(dirname $prefix) && split -d -n r/$slices $in $prefix
|
|
|
|
# wiki2text is a tool I wrote using Nim 0.11, which extracts plain text from
|
|
# Wikipedia dumps obtained from dumps.wikimedia.org. The code is at
|
|
# https://github.com/rspeer/wiki2text.
|
|
rule wiki2text
|
|
command = bunzip2 -c $in | wiki2text > $out
|
|
|
|
# To tokenize Japanese, we run it through Mecab and take the first column.
|
|
rule tokenize_japanese
|
|
command = mecab -b 1048576 < $in | cut -f 1 | grep -v "EOS" > $out
|
|
|
|
# Process Chinese by converting all Traditional Chinese characters to
|
|
# Simplified equivalents -- not because that's a good way to get readable
|
|
# text, but because that's how we're going to look them up.
|
|
rule simplify_chinese
|
|
command = python -m wordfreq_builder.cli.simplify_chinese < $in > $out
|
|
|
|
# Tokenizing text from Twitter requires us to language-detect and tokenize
|
|
# in the same step.
|
|
rule tokenize_twitter
|
|
command = mkdir -p $$(dirname $prefix) && python -m wordfreq_builder.cli.tokenize_twitter $in $prefix
|
|
|
|
rule tokenize_reddit
|
|
command = mkdir -p $$(dirname $prefix) && python -m wordfreq_builder.cli.tokenize_reddit $in $prefix
|
|
|
|
# To convert the Leeds corpus, look for space-separated lines that start with
|
|
# an integer and a decimal. The integer is the rank, which we discard. The
|
|
# decimal is the frequency, and the remaining text is the term. Use sed -n
|
|
# with /p to output only lines where the match was successful.
|
|
#
|
|
# Grep out the term "EOS", an indication that Leeds used MeCab and didn't
|
|
# strip out the EOS lines.
|
|
rule convert_leeds
|
|
command = sed -rn 's/([0-9]+) ([0-9.]+) (.*)/\3,\2/p' < $in | grep -v 'EOS,' > $out
|
|
|
|
# To convert the OpenSubtitles frequency data, simply replace spaces with
|
|
# commas.
|
|
rule convert_opensubtitles
|
|
command = tr ' ' ',' < $in > $out
|
|
|
|
# To convert SUBTLEX, we take the 1st and Nth columns, strip the header,
|
|
# run it through ftfy, convert tabs to commas and spurious CSV formatting to
|
|
# spaces, and remove lines with unfixable half-mojibake.
|
|
rule convert_subtlex
|
|
command = cut -f $textcol,$freqcol $in | tail -n +$startrow | ftfy | tr ' ",' ', ' | grep -v 'â,' > $out
|
|
|
|
rule convert_jieba
|
|
command = cut -d ' ' -f 1,2 $in | grep -v '[,"]' | tr ' ' ',' > $out
|
|
|
|
rule counts_to_jieba
|
|
command = python -m wordfreq_builder.cli.counts_to_jieba $in $out
|
|
|
|
|
|
# Convert and clean up the Google Books Syntactic N-grams data. Concatenate all
|
|
# the input files, keep only the single words and their counts, and only keep
|
|
# lines with counts of 100 or more.
|
|
#
|
|
# (These will still be repeated as the word appears in different grammatical
|
|
# roles, information that the source data provides that we're discarding. The
|
|
# source data was already filtered to only show words in roles with at least
|
|
# two-digit counts of occurences.)
|
|
rule convert_google_syntactic_ngrams
|
|
command = zcat $in | cut -f 1,3 | grep -v '[,"]' | sed -rn 's/(.*)\s(...+)/\1,\2/p' > $out
|
|
|
|
rule count
|
|
command = python -m wordfreq_builder.cli.count_tokens $in $out
|
|
|
|
rule count_langtagged
|
|
command = python -m wordfreq_builder.cli.count_tokens_langtagged $in $out -l $language
|
|
|
|
rule merge
|
|
command = python -m wordfreq_builder.cli.merge_freqs -o $out -c $cutoff -l $lang $in
|
|
|
|
rule merge_counts
|
|
command = python -m wordfreq_builder.cli.merge_counts -o $out -c $cutoff $in
|
|
|
|
rule freqs2cB
|
|
command = python -m wordfreq_builder.cli.freqs_to_cB $in $out -b $buckets
|
|
|
|
rule cat
|
|
command = cat $in > $out
|
|
|
|
# A pipeline that extracts text from Reddit comments:
|
|
# - Unzip the input files
|
|
# - Select the body of comments, but only those whose Reddit score is positive
|
|
# (skipping the downvoted ones)
|
|
# - Skip deleted comments
|
|
# - Replace HTML escapes
|
|
rule extract_reddit
|
|
command = bunzip2 -c $in | $JQ -r 'select(.score > 0) | .body' | fgrep -v '[deleted]' | sed 's/>/>/g' | sed 's/</</g' | sed 's/&/\&/g' > $out
|
|
|